Healthcare revenue cycle analytics isn’t just another dashboard—it’s how top-performing hospitals and clinics uncover revenue leaks, denial risks, and billing inefficiencies before they cost real money. In an era where even a 1% drop in clean claims impacts margins, the ability to act on timely insights is no longer optional.
In this blog, we explore the strategies that drive better results—and the missteps that often lead to delays, denials, or worse: lost reimbursements.
What Is Healthcare Revenue Cycle Analytics?
Healthcare revenue cycle analytics refers to the process of evaluating data generated throughout the revenue cycle—from scheduling and registration to claim submission and final reimbursement. The goal is to monitor KPIs, identify problem areas, and improve collections across every stage.
It provides visibility into billing operations that otherwise go unnoticed, helping providers streamline workflows and reduce claim rework. Explore more in our in-depth guide on how revenue cycle analytics supports RCM improvement.
What’s Actually Working in Healthcare Revenue Analytics Today
Analytics tools are most valuable when they help providers act—not just observe. Here’s what’s proving effective in real-time environments:
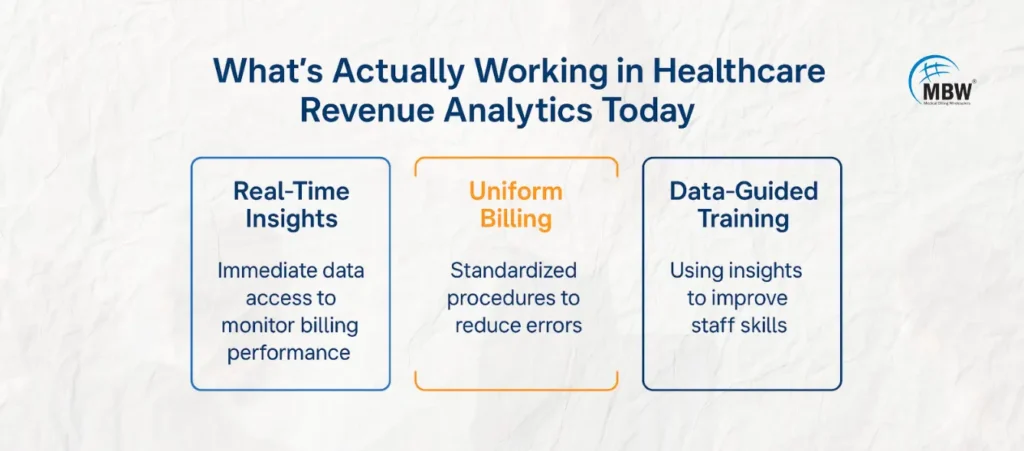
1. Spotting Denial Patterns Before They Affect Revenue
The ability to flag trends in denied claims has been a turning point for many revenue cycle teams. By analyzing payer responses in real time, billing departments can identify root causes—like coding mismatches or documentation gaps—and take corrective action before the problem grows.
A 2024 report by RevCycleIntelligence shows that denial rates dropped by 15% in practices that implemented automated denial analytics tools.
2. Closing Gaps in Patient Data That Delay Payments
Most delays start with bad data. Healthcare revenue cycle analytics helps highlight missing or mismatched patient information—especially around insurance eligibility or coverage details—that would otherwise cause a rejection.
By proactively flagging incomplete data before a claim is submitted, providers not only reduce rework but also improve clean claim rates, resulting in faster payments.
3. Turning Billing Metrics Into Actionable Team Improvements
Analytics isn’t just about numbers—it’s about people. By analyzing trends in staff performance, recurring errors, or undercoded procedures, billing managers can provide targeted coaching and process fixes that directly impact claim quality.
To learn how analytics can support better staffing decisions, check out our RCM analytics services for healthcare organizations.
What Works vs What Fails?
What Works Well | What Fails Frequently |
---|---|
Real-time alerts and monitoring | Ignoring repeat denial patterns |
Consistent billing practices | Poor insurance eligibility checks |
Regular staff training using data insights | Relying on outdated billing software |
Predictive analytics for denial prevention | Delaying action on claim denials |
Real-time denial management systems | Failing to update coding practices |
What Fails in Healthcare Revenue Cycle Analytics
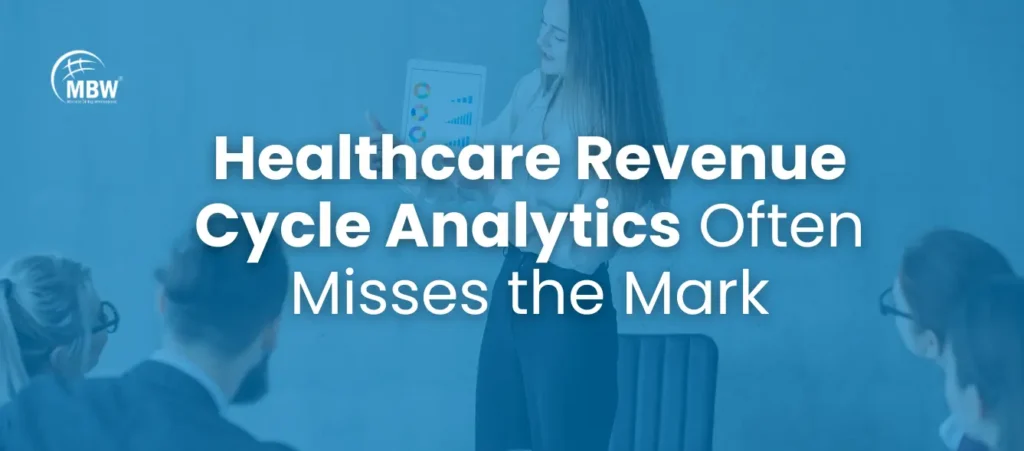
1. Ignoring Denial Trends
A significant failure in revenue cycle analytics occurs when healthcare organizations overlook repeat denial trends. By not acting on analytics insights, claims issues persist, leading to lost revenue.
2. Missed Insurance Eligibility Checks
Failing to check insurance eligibility before submitting claims is a common mistake that can result in denials. Healthcare revenue cycle analytics can catch eligibility issues before submission, reducing the risk of claim rejections.
3. Overreliance on Outdated Systems
Many healthcare organizations still use legacy billing systems, which can’t provide the real-time analytics necessary to prevent errors. These outdated systems often lead to inefficiencies, such as delayed claims or missed payments.
“Data is the new oil, and analytics is the combustion engine that powers success in the revenue cycle.”
The Power of Predictive Analytics in Revenue Cycle Management
Predictive analytics is a game-changer in revenue cycle management. By analyzing historical billing data, predictive models can forecast problems before they occur. For example, predictive models can identify which claims are at a high risk of being denied or delayed. This foresight allows the revenue cycle team to proactively address these claims before submission.
Example: A predictive model could flag claims that are more likely to be denied due to incorrect coding or insufficient documentation. The team can then correct these issues before submission, minimizing the chances of denials.
“Predictive analytics doesn’t just provide insights; it empowers teams to act before problems occur, driving greater financial success.”
How AI and Machine Learning Are Revolutionizing Revenue Cycle Analytics
AI and machine learning enable healthcare systems to process large volumes of billing data faster than manual methods.
These technologies identify complex claim patterns often missed through traditional review.
AI can automatically:
Flag coding errors
Detect duplicate claims
Streamline claim submissions
Machine learning can analyze claims for trends based on payer behavior, helping billing teams make timely adjustments.
Example: If AI identifies a high denial rate from a specific payer, teams can investigate and resolve the root issue quickly.
“AI and machine learning are transforming the revenue cycle by automating tedious tasks and providing deeper, more accurate insights into billing processes.”
The Power of Real-Time Denial Tracking in Reducing Revenue Delays
Real-time denial management systems are essential for maintaining a healthy cash flow. With real-time data, denial trends can be identified and resolved almost immediately, reducing the time spent in the appeals process and improving overall revenue cycle efficiency.
Example: If a claim is denied due to an eligibility issue, real-time alerts allow the billing team to quickly resolve the issue and resubmit the claim without waiting for the payer to process it.
“Real-time denial management systems minimize delays, ensuring claims are processed faster and reducing revenue loss.”
Using Data Analytics to Improve Billing Compliance in Healthcare
Healthcare regulations like ICD-10 and CPT coding guidelines change frequently.
Data analytics help identify areas where billing or coding practices are not compliant.
Organizations can proactively correct errors before audits or penalties occur.
Analytics ensures teams follow updated guidelines, avoiding unnecessary denials.
Example: A coding discrepancy flagged by analytics can be corrected before the claim is sent, improving approval chances.
“Data-driven insights not only enhance billing accuracy but also ensure compliance with the latest healthcare regulations, minimizing the risk of audits and penalties.”
Conclusion
Healthcare revenue cycle analytics is a vital tool for improving the efficiency and profitability of healthcare organizations. By focusing on real-time monitoring, predictive analytics, and cross-departmental collaboration, healthcare providers can significantly reduce errors, denials, and missed payments. However, success lies in knowing what works—and avoiding what fails.
To truly optimize your revenue cycle, it’s essential to invest in the right technology, staff training, and data-driven strategies. Organizations that stay ahead of the curve with up-to-date systems, real-time monitoring, and advanced analytics are better positioned to thrive in an increasingly complex healthcare environment.
Frequently Asked Questions (FAQs)
What is healthcare revenue cycle analytics?
Healthcare revenue cycle analytics involves using data to assess and optimize the billing process from patient registration to final payment. It helps identify areas for improvement and increase the efficiency of revenue collection.How can predictive analytics help in healthcare billing?
Predictive analytics can forecast trends in claim denials, payment delays, and other issues, allowing teams to address these problems before they impact cash flow.Why is real-time monitoring essential for revenue cycle management?
Real-time monitoring helps healthcare organizations spot and address issues as they happen, improving cash flow and reducing the chances of claim denials and delays.How does AI improve healthcare revenue cycle management?
AI improves revenue cycle management by automating repetitive tasks, such as flagging coding errors and identifying denial patterns. It also provides deeper insights, improving decision-making and operational efficiency.